Traditional vs. algorithmic tenant screening: What’s really the difference?
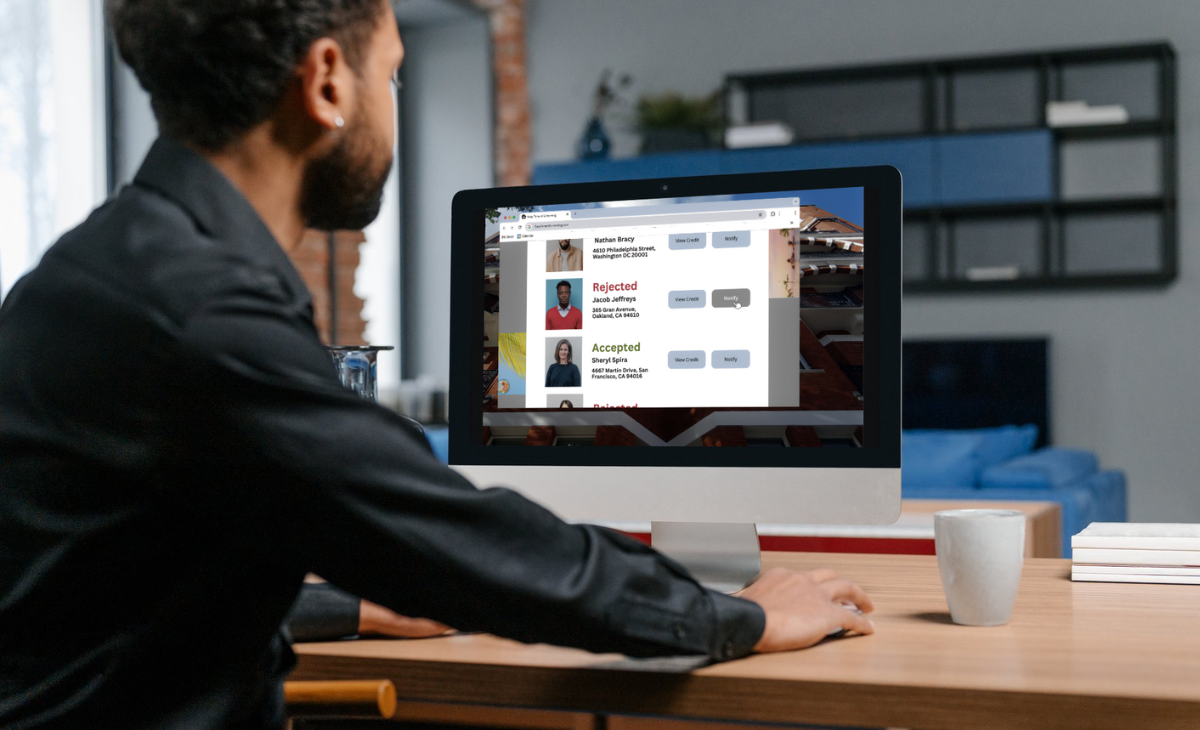
A lawsuit filed in Florida alleged that the tenant-screening algorithms used by a large local landlord discriminate against Black rental applicants.
Meanwhile, housing voucher recipients in Massachusetts recently sued SafeRent, arguing that their algorithmic tenant screening program (the “SafeRent Score”) disproportionately discriminated against them. And it didn’t go unnoticed that these recipients are also disproportionately Black and Latinx.
The federal government is catching on too.
Like many advances in the tech industry, algorithmic tenant screening was pitched as a solution to an old problem. This “objective” replacement was supposed to eliminate biased traditional tenant screening, protecting landlords from lawsuits and streamlining their process while promising tenants a fair shot at housing.
That’s clearly not what happened. So what’s really the difference between algorithmic and traditional tenant screenings?
Hold on; what’s tenant screening in the first place?
Tenant screening is a process through which landlords decide whether or not they think someone will be a good, on-time paying renter. A prospective renter turns in an application with their information—name, contact information, income, living/rental history, and social security number.
Next, landlords typically run a credit check and a background check, which ideally reflect a prospective renter’s trustworthiness and ability to pay rent. Some landlords might opt for rental history and income verification to provide additional insight and context. Then they decide whether or not to approve the renter’s application.
There’s a lot of room for bias in this situation—from issues with credit reports to issues with landlords themselves. The Fair Housing Act and Fair Credit Reporting Act attempted to limit bias and discrimination in housing and beyond, but it continues on—even with algorithms.
Both are biased—but only one is hard-coded that way
There’s a dangerous assumption that algorithms aren’t biased, or at least not as much as human beings. But algorithms aren’t as objective as they’re made out to be. They’re created by biased humans and fed biased data—but then used as if they’re not biased themselves.
For instance, Black people in the United States are five times more likely to be arrested than white people, even when they’re not convicted of any crime. Nevertheless, an arrest alone can be fed into a tenant screening algorithm, resulting in the applicant getting rejected.
The inclusion of this data point in a tenant screening is within itself an example of the bias of the people who designed the tool. Why is arrest an important factor in screening applicants? Would someone from a community that is disproportionately arrested without cause include that data point?
Landlords and rental companies can use similarly biased factors in traditional tenant screening, but that’s an individual choice. When using algorithmic tenant screenings, that choice is automated at scale.
This is becoming a growing issue as large companies swallow up more and more rental properties—even single-family homes. They’re able to do this thanks to automated decision-making tools that don’t “need” as much personal oversight.
These algorithms’ decisions don’t come with context. They don’t even say why exactly the renter was rejected, so neither the landlord nor the applicant can see if the data inputs are possibly incorrect or misleading. That makes it impossible to correct and/or contextualize these inputs.
No transparency and no accountability
With traditional tenant screening, there’s at least some transparency regarding what data is being collected and why an application might be rejected. The renter knows what information they gave the landlord and the landlord knows what information they collected.
When it comes to algorithmic tenant screening, renters rarely know what software was used to evaluate their application. Even if they did, it wouldn’t help much. In 2022, TechEquity landscaped the rental screening industry but found that many of the specifics of how algorithmic tenant screening companies operate are hidden behind proprietary products and claims of trade secrets.
The landlords and companies using the software don’t even know exactly what data is being collected and how it’s being weighed to approve or reject rental applications. So how do we assess for bias? How can renters know if they’re being discriminated against? Who should be held accountable? How can anyone even be held accountable?
These are difficult questions to answer when it comes to holding people accountable for any form of bias. However, with the black-box nature of algorithmic tenant screening, answers become exponentially harder to find.
There are no consistent, concrete, accountable, or agreed-upon standards at a regulatory—or even industry—level to ensure that the data being used to build the algorithm is appropriately collected, trained, secured, and does not create a discriminatory outcome. Additionally, the companies that make algorithmic tenant screening software say they’re not subject to the Fair Housing Act because their scores only advise landlords—they don’t make the decisions.
As for the landlords, they don’t know what’s going on behind these algorithms. So how can they be held accountable for algorithmic discrimination?
Who’s left holding the bag?
When you have biased hard-coded into opaque algorithms that have no incentive to be transparent, who do you think is going to get the short end of the stick? Renters.
Renters of color already have a worse time in the current rental market. A study found that in every major U.S. city, property managers were less likely to respond to prospective Black and Latinx tenants when they inquired about open listings.
TechEquity’s forthcoming research into tenant screening practices revealed that landlords charging lower rents are more likely to rely on third-party analysis than landlords overall. This means that low-income people are also disproportionately impacted by algorithmic tenant screening. Pair that with the fact Black and Hispanic adults are more likely to stuck with low incomes, and you get a recipe for class and racial discrimination.
It all adds up: the most vulnerable are the most impacted and the most discriminated against. They then don’t have access to the resources to advocate for themselves in the face of discrimination.
What we can do about it
That’s why we need to act now. We need to understand these models and establish guardrails that require bias reduction and transparency. We need to create regulations that prevent harm and create avenues for those harmed to hold companies accountable.
In alignment with our AI Policy Principles, we’re supporting AB 2930 in California. AB 2930 requires developers and users of Automated Decision-Making Tools (ADTs) to conduct and record an impact assessment. The data reported must include an analysis of potential adverse impact on the basis of race, color, ethnicity, sex, or any other classification protected by state law.
On the federal level, we’re encouraged by the Department of Housing and Urban Development (HUD), which issued Guidance on the Application of the Fair Housing Act to the Screening of Applicants for Rental Housing. This guidance clarifies the responsibilities of housing providers and screening companies to ensure that the use of this technology doesn’t lead to discriminatory housing decisions that violate the Fair Housing Act.
Sign up for our newsletter to stay up-to-date on the news around algorithmic tenant screening—and to be notified when we publish our new research report.