The AI supply chain, explained
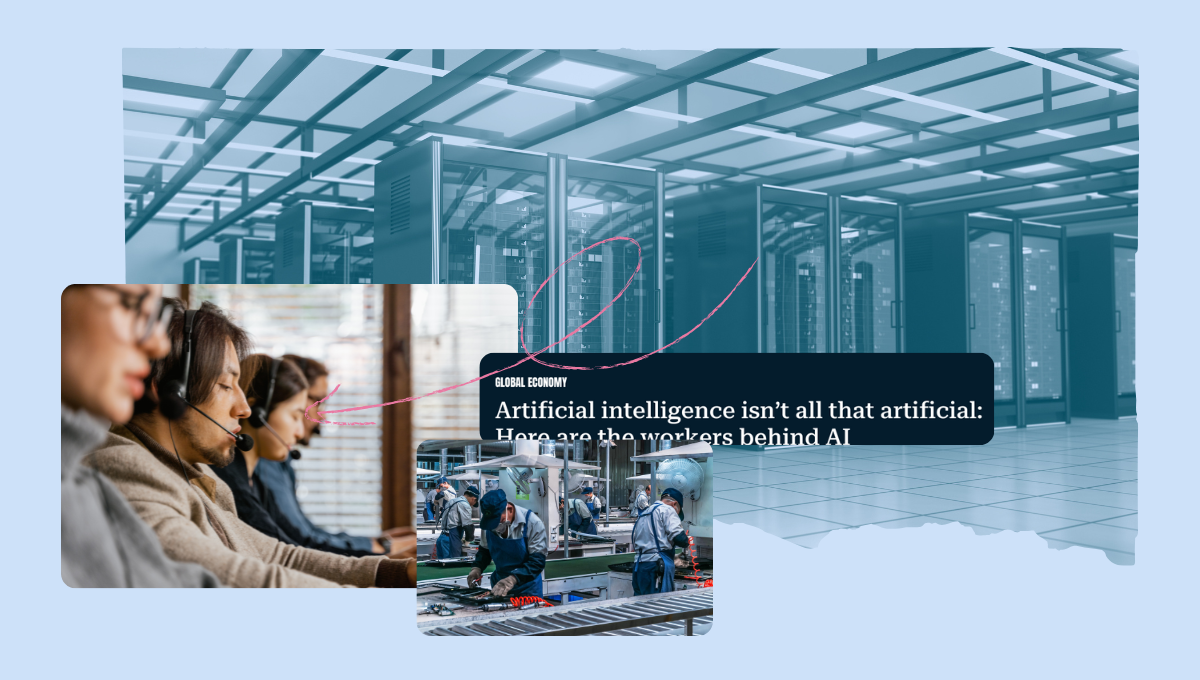
In 2005, Amazon Mechanical Turk (MTurk for short) was created—a service that allows anyone to hire workers from around the world to carry out large numbers of ‘micro’ tasks. At the time, CEO Jeff Bezos called MTurk ‘artificial artificial intelligence’. Behind the facade of the machine were scores of humans performing small and repetitive tasks that are difficult or even impossible for computers to perform.
Nearly two decades on, tech companies have advanced a new hype cycle related to artificial intelligence that they claim can save people from the drudgery of monotonous tasks, make them safer and more productive, and make their day-to-day lives easier, all while saving businesses time and money. But behind the facade of automation, AI continues to be powered by workers around the world connected by supply chains.
What is the AI Supply Chain?
Although the term “supply chain” has been used to describe the raw material and processes that go into the production and distribution of a commodity, the proliferation of the internet and communication technology has made it even easier and more profitable for companies to distribute work that builds these technologies around the world.
Supply chains that produce commodities such as clothes and groceries have become visible and less opaque due to dedicated research, advocacy, and worker organizing. Decades of garment worker organizing, combined with consumer and investor pressure, along with the activism of groups like United Students Against Sweatshops, pushed apparel brands to be more transparent about where they source products. Organizations like the Worker Rights Consortium help enforce labor standards in the industry, conduct worker-centered investigations, and implement remedies when garment workers experience violations of their rights. The same is not yet true for AI systems, although they are also built upon networks of human labor spread across the world.
When they receive a tangible benefit, such as being able to scroll through their social media accounts without being exposed to traumatizing content or using an AI assistant to complete a presentation, consumers interact with the front end of the purportedly automated technology, which provides no indication of how workers are involved in generating the service being provided.
In addition to the vast amount of unpaid human labor in the form of content (text, images, sound, videos) that is used to train AI systems, paid human labor is integrated into the development of such systems through supply chains in ways that are essential and yet often obscured by tech companies. Human labor is, in fact, central to developing, training, testing, and maintaining AI systems.
Labor in the AI Supply Chain
Discussions of AI tend to focus on how it might impact our jobs, whether through job loss or augmentation. Both these narratives leave out the centrality of labor to the very functioning of AI systems. AI tools exist because of a vast global supply chain that includes everything from mineral extraction to manufacturing, shipping, data storage, and handling e-waste and connects workers and communities across continents. The segment of this supply chain that makes the datasets and trains the algorithms that form the basis of AI—referred to as “data work”—provides a helpful case study of how work is sourced to fuel the current AI boom.
While it might be easy to imagine that engineers are necessary to write the algorithms that produce AI, tech companies provide little transparency about the vast number of data workers who build, populate, train, and maintain these systems. The globally distributed nature of this workforce is also only now becoming apparent as more workers are starting to speak out. AI companies are largely concentrated in North America and Europe, whereas AI labor flows primarily from the Global South.
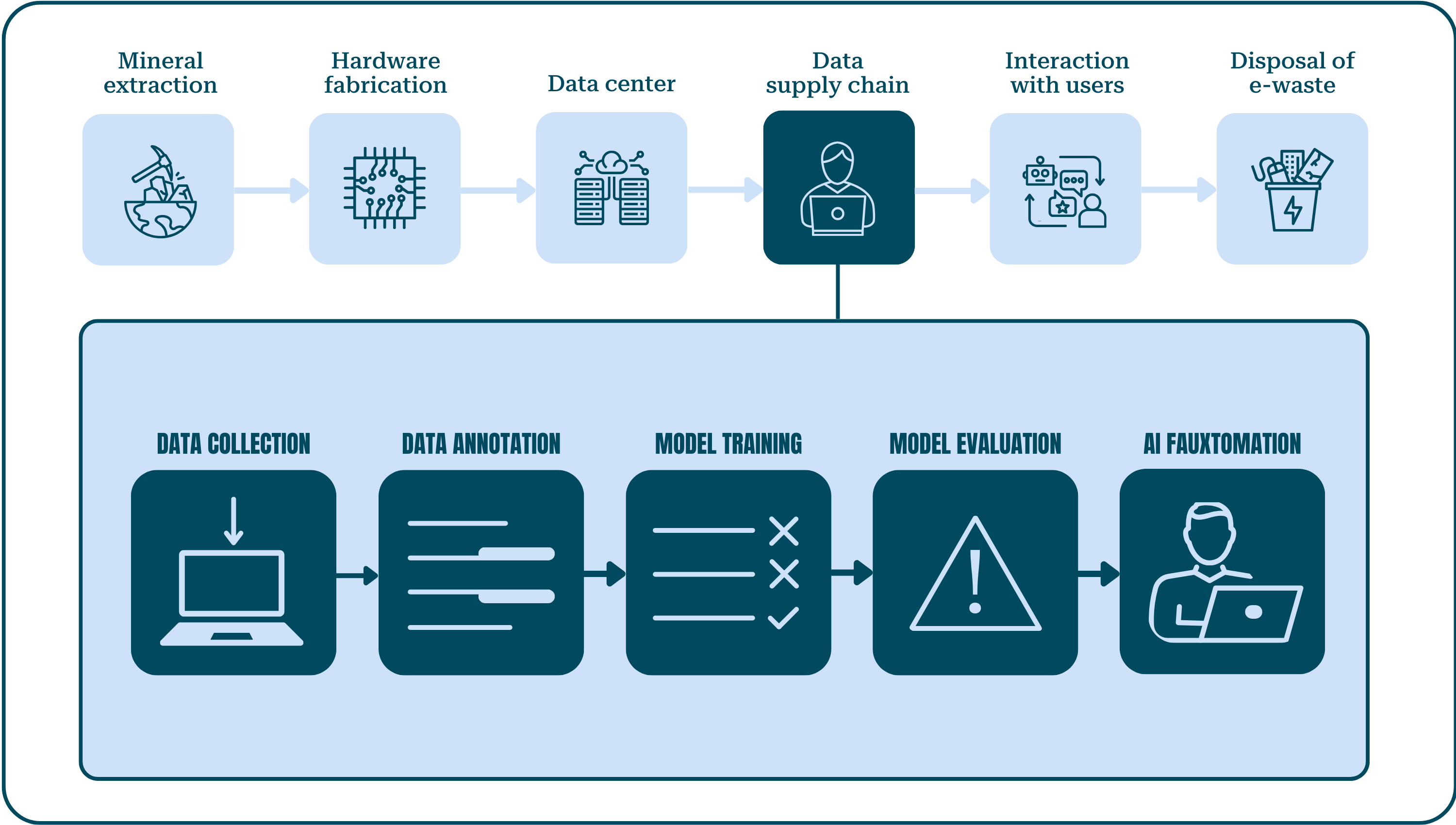
What do data workers in the AI supply chain do? Before answering this question, it is important to underline the complexity of AI production processes and the interconnected and iterative nature of different types of data work. What follows is a high-level sketch that reveals data workers to be the keystone of the AI supply chain rather than a detailed description of the myriad intricate ways in which workers enable AI production.
Data Collection
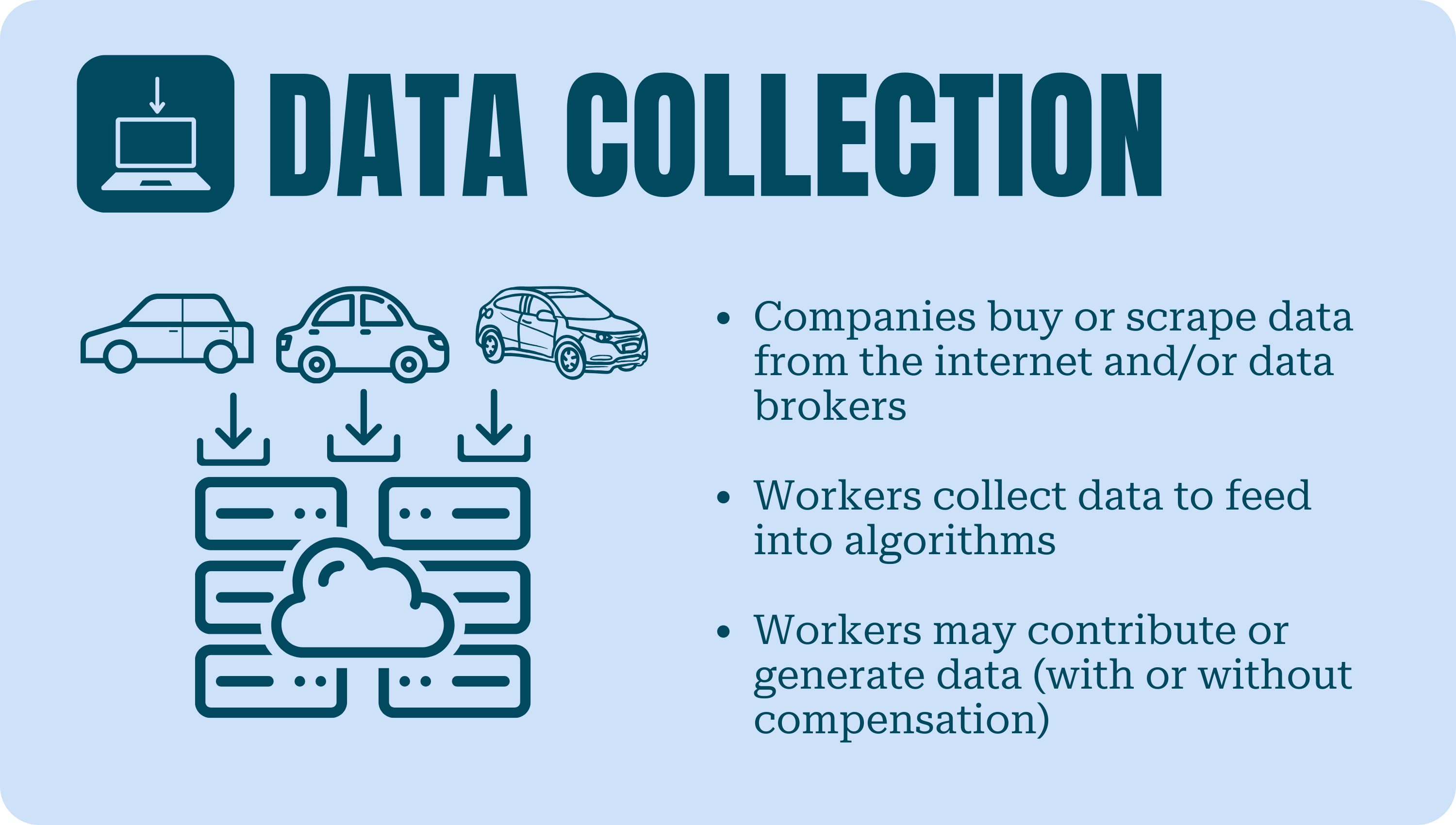
Although much of the data that is used to build and train AI models is collected through the purchase of datasets, web-scraping, or open-source repositories, data workers are occasionally engaged in collecting data or even contributing data. Data workers might be tasked with generating voice data, allowing companies to expand their databases of local languages, dialects, and idioms.
In another instance, data workers who were also small business owners contributed datasets comprising financial, customer, and inventory data. Workers were reportedly compensated for a few of the datasets before the project was terminated with no advance notice, leaving workers concerned about their business data and fighting to be paid. While the lack of transparency and having to work in the absence of a larger context is a problem at all stages of data work, it is particularly fraught for workers who contribute personal data.
Data Annotation
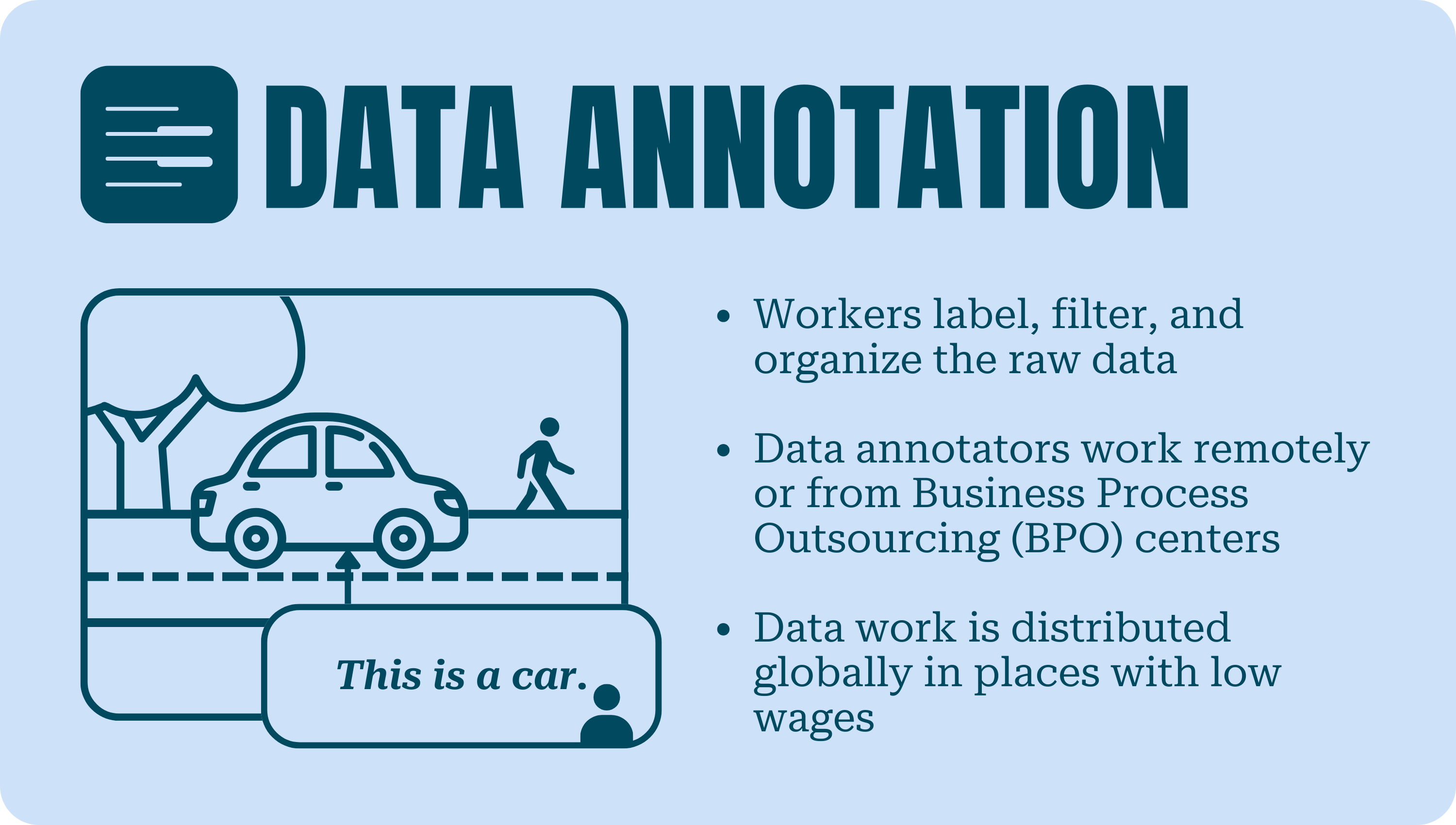
AI systems are usually built on supervised machine learning algorithms where supervision refers to feeding an algorithm vast quantities of high-quality, annotated data that is produced by data workers, often working remotely from their own homes via online platforms or from Business Process Outsourcing (BPO) centers. Data that is scraped from the web and from social media platforms and has already been moderated by a globally dispersed and largely contract workforce of content moderators is then labeled, filtered, and organized by data workers for AI systems to learn how to infer outputs correctly for a wide variety of use cases.
Although there are some indications that tech companies are increasingly seeking out people who have niche expertise in specific fields to enrich data, data annotation is often seen as a low-level input to AI systems. In order to reduce the costs incurred to carry out these essential tasks, data work is distributed around the world, specifically to workers in the Global South, where poorly enforced labor law regimes and low labor costs intersect.
However, the consistent demand for high-quality annotated data and the far-reaching negative consequences of unlabeled or incorrectly annotated data show that the devaluation of data annotation is not grounded in the specificities of the AI system, but is rather a means to justifying low remuneration in this section of the supply chain. The regular use of quality assurance processes also underlines the necessity of accurate data annotation.
Model Training
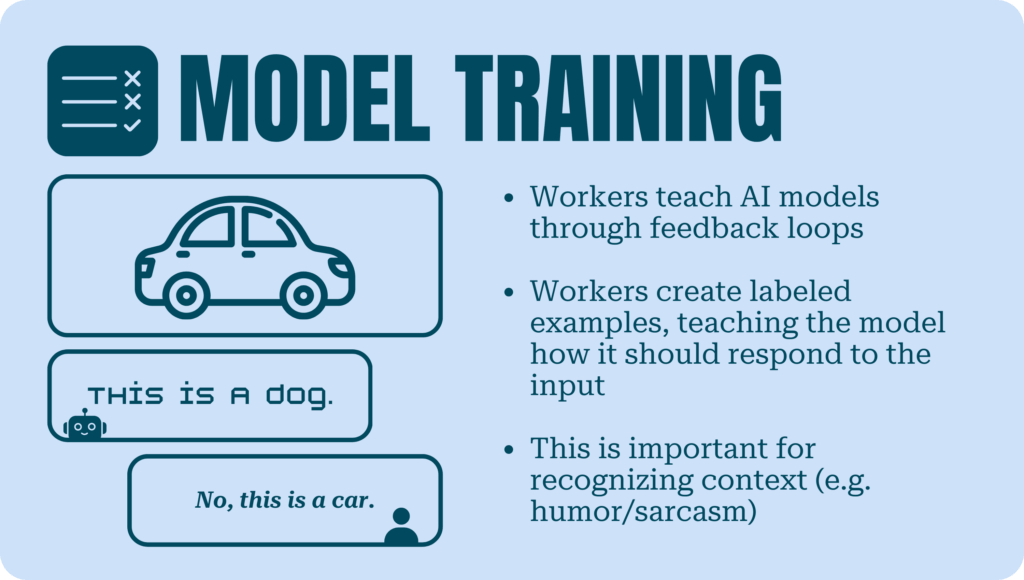
Workers also play a central role when AI systems are based on unlabeled data. Through processes such as RLHF (reinforcement learning from human feedback) and SFT (supervised fine-tuning), workers teach AI systems to output the right response through feedback loops that give positive and negative signals to the algorithm.
Training by humans is also essential to teach AI systems how to perform tasks whose goals are difficult to specify. For instance, a large language model may be unable to identify emotions or biases encoded in text, respond to a prompt in a way that is expected by the user, or go beyond a literal translation of a piece of text. In such situations, human experts create labeled examples, demonstrating how the LLM ought to respond in various use cases.
Model Evaluation
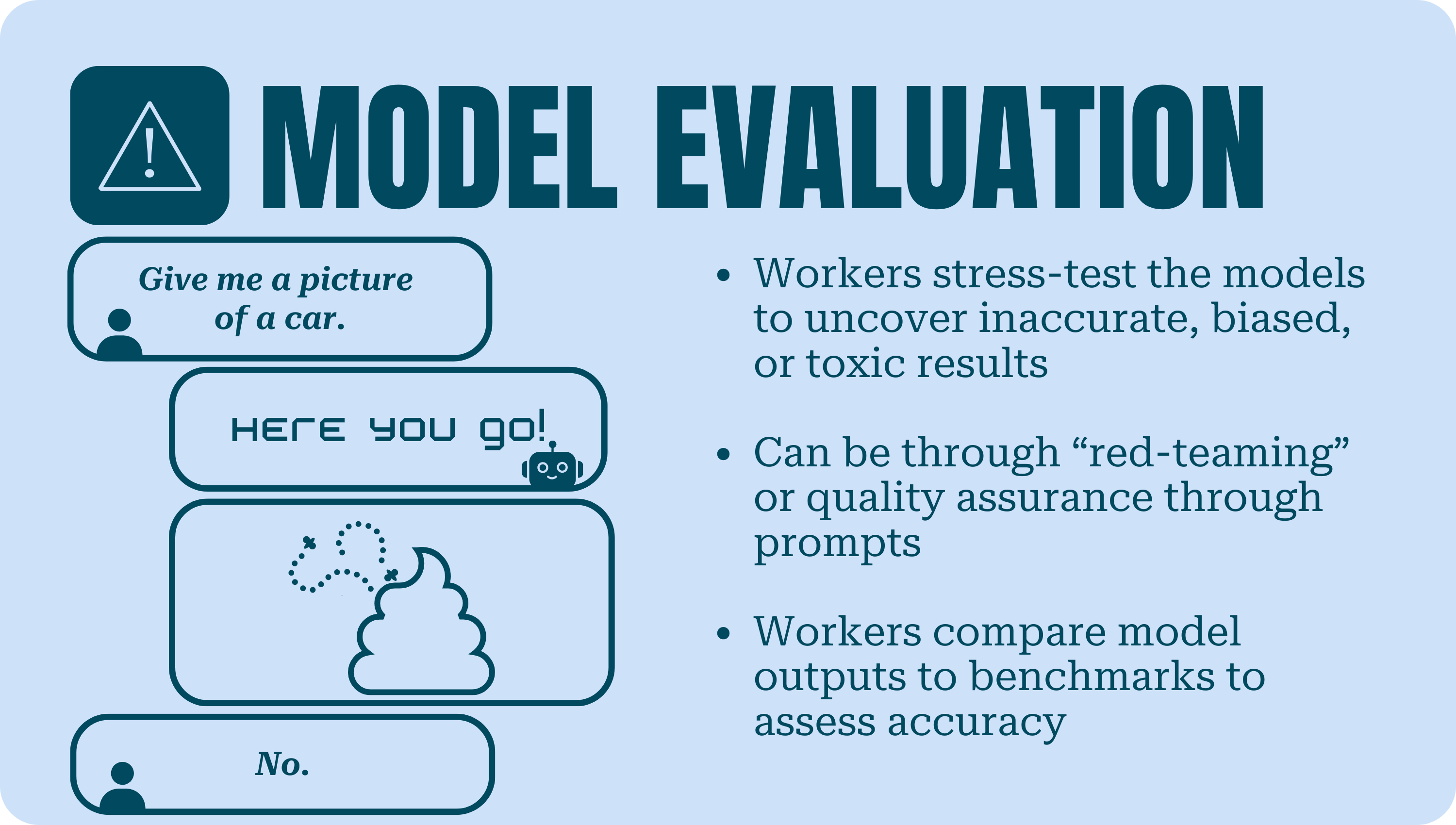
Before an AI system is deployed and even when it’s in use, continuous testing, also referred to as validation or verification, is carried out by workers through prompt-response processes. This can include processes such as red-teaming, where workers stress test AI models through provocative prompts to uncover potentially biased, inaccurate, hallucinatory (invented by the AI system), or toxic content, as well as checking a model’s accuracy by comparing its output to benchmarked standards. Such testing and maintenance work makes AI systems better, keeps them secure, and limits their ability to cause widespread harm. Programmers in countries such as India, Pakistan, Brazil, and Indonesia who work at a fraction of the cost of similar workers in the Global North have been found to be integrated into the AI supply chain to carry out this critical phase.
AI Fauxtomation
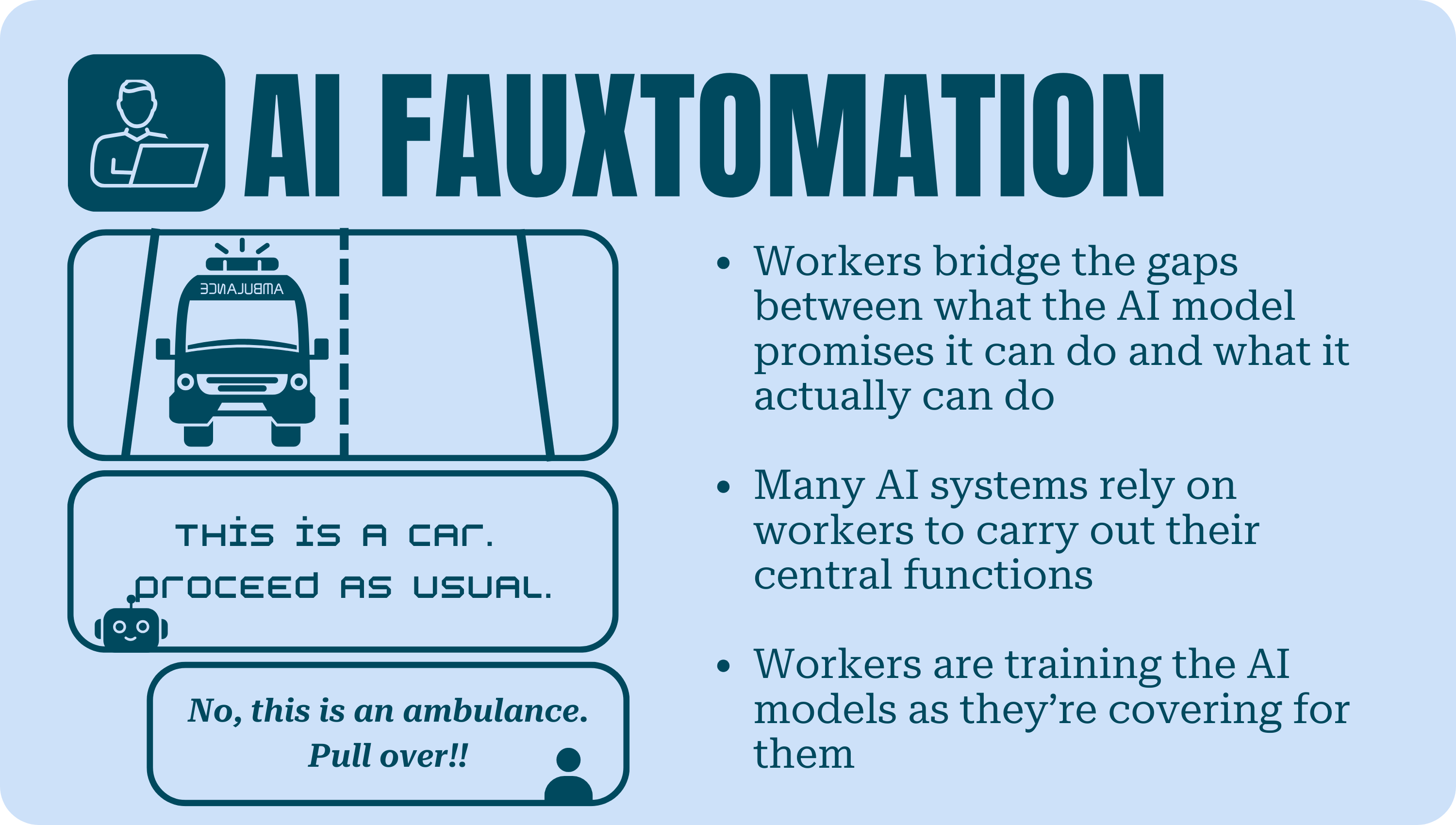
Although workers are able to train AI systems to get better at mimicking human responses, these systems are still far from perfect, leading to another significant category of interventions by workers. By bridging the gaps between what AI’s proponents claim it can do and what it can actually do, workers engage in ‘AI impersonation’ or ‘fauxtomation.’
Systems that claim to be automated have been found to rely on workers to carry out their central functions. From AI assistants who turned out to be human to self-checkout stores that were managed remotely by workers in India and ‘autonomous’ vehicles that are being guided by technicians hundreds of miles away, evidence abounds that AI systems sustain the illusion of being automated by depending heavily on human labor. Workers who are impersonating AI are at the same time generating data that is used to train and perfect the system they are impersonating. However, improvements in AI models are predicted to reduce but not eliminate dependence on human labor—the human in the loop is an incontrovertible, structural feature of AI models.
Harms in the AI Supply Chain
Despite the essential roles they play in enabling every stage of an AI system, the conditions under which workers labor in the AI supply chain have been found to be remarkably poor. In one instance, researchers found that while an AI model is engineered and managed in France, tasks such as data annotation and training, which require more work hours, are outsourced to Madagascar and Indonesia, which offer very low labor costs. While the scientists and engineers in France represent the visible and well-remunerated part of the AI supply chain, the data workers in Madagascar and Indonesia remain poorly paid and largely invisible, hidden behind the claim of automation inherent to AI systems.
In another example that highlights the exploitative nature of AI supply chains and the harms they can cause to workers, in order to train ChatGPT, OpenAI outsourced labeling of toxic content to workers in Kenya who were reportedly paid less than $2 per hour to trawl through vast quantities of traumatizing text, images, and videos. Thus, workers preventing AI systems from generating harmful content do so at great risk to their own mental health.
Besides the psychological impacts of being exposed to such content, workers also have to contend with the precarity built into data work. The big tech companies at the top of the AI supply chain often source data work through layers of contractors, vendors, and platforms. These companies frequently shift work around the world, leading to loss of livelihoods with little or no notice. Workers on digital platforms have found themselves suddenly laid off, locked out of their accounts, or even denied payment for work already completed. With little recourse to legal protection and no clear channels of communication with these companies, workers are left to fend for themselves. These examples show that the poor quality of data worker jobs is a common thread that connects workers all along the AI supply chain.
What We’re Doing About It
Companies have long outsourced labor-intensive work to the Global South, to the detriment of not only local workers but also workers beyond our borders who are paid paltry wages for insecure work that can be physically and mentally demanding. We recognize that the centrality of data workers to the functioning of AI systems can act as a powerful lever of influence while their shared conditions of work create a basis for global networks of solidarity. To make data worker jobs better for workers across the AI supply chain, we can:
- Work with partners to advance labor rights in California and in the US to ensure good quality jobs for data workers, creating a decent work floor that can build worker power and serve as a benchmark for workers everywhere.
- Create guardrails that would prevent US-based companies from instituting exploitative labor practices globally.
- Build solidarity with workers throughout the supply chain through a global coalition of labor advocates that acknowledges the importance of data work in creating better livelihoods for workers in the U.S. and beyond our borders.
- Engage with state, national, and global regulators at the intersection of workers’ rights and technology to identify best practices in regulating data work across borders.
Join us
Do you work in this space and want to collaborate with us? We’d love to hear from you; you can get in touch with us here. You can also sign up for our newsletter or follow us on social media to stay up-to-date with info and action items.