Screened Out of Housing – In a nutshell
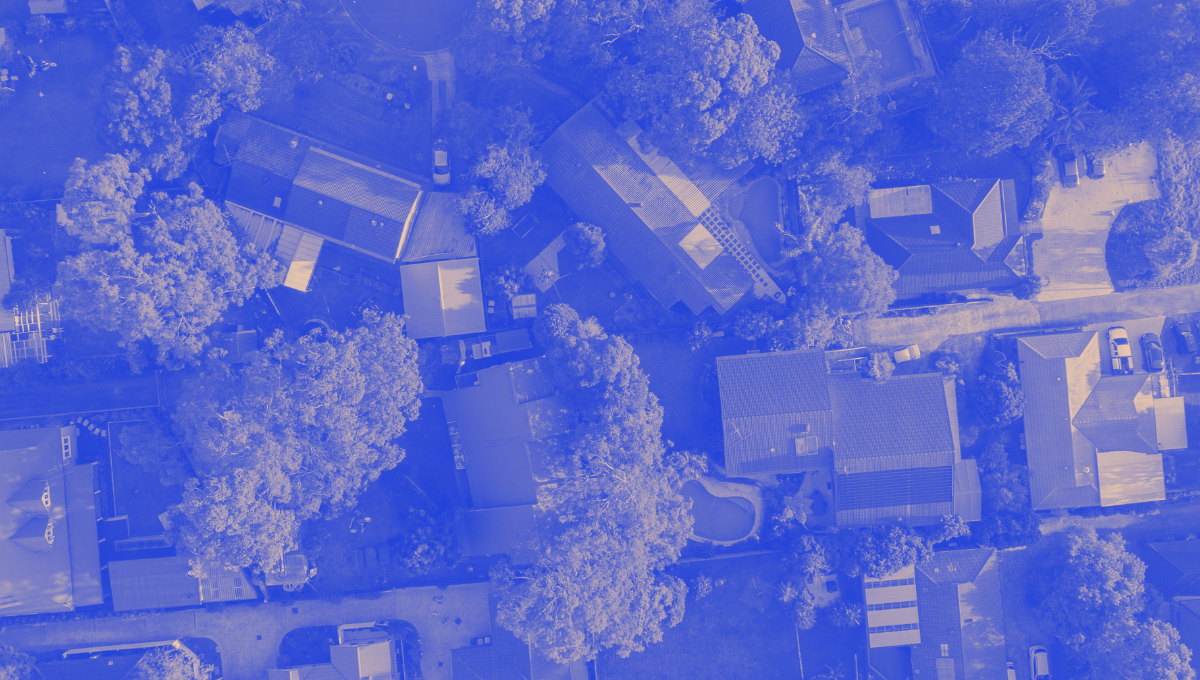
When you apply for rental housing, your potential landlord will conduct some sort of tenant screening process through which they decide whether or not they think you’ll be a good renter. They’ll ask you for basic personal information, proof of income, references, and consent for the landlord to pull various background reports, including rental history, social security number, credit score, and criminal history.
This process is fraught with bias, from racist credit scoring to—well, racist landlords.
As AI floods every sector, automated decision-making and artificial intelligence systems are coming for tenant screening too. We already know that AI systems have a tendency to discriminate against already-vulnerable communities, in healthcare, the criminal legal system, and more. Now, when you apply for housing, it could very well be that some black-box AI is deciding whether or not you’re worthy of getting a home.
Housing advocates around the country have been calling for more equitable practices when it comes to legacy tenant screening. The problem that algorithmic tenant screening introduces, however, is that it outsources those decisions—and along with it, the bias and responsibilities that come with it.
Black-box housing decisions
With AI-powered tenant screening, everyone is left in the dark. Tenants don’t know what’s behind a landlord’s decision to approve or deny their housing application. Landlords who increasingly rely on algorithms within third-party software to provide them with screening recommendations don’t have a clear understanding of what went into that outcome. The opacity creates big gaps for enforcing consumer and housing protections.
We need to understand how these algorithms work and how they’re being used in housing decisions. Only then can we craft policy that centers the needs of renters and protects them against encoded bias.
Unpacking the black box
We wanted to shed light on this opaque industry and how it impacts renters. So, we at TechEquity partnered with Wonyoung So, a Ph.D. candidate at the Department of Urban Studies and Planning at MIT to develop a survey for landlords and renters in California asking about their use and understanding of tenant screening AI.
We received responses from over 1,000 renters and 400 landlords in California. Why California? California is the state with the second-largest share of renter households, making the joint surveys the most extensive insights to date into how landlords work with CRAs (Consumer Reporting Agencies, for example, Experian or Equifax) to make rental decisions.
What we learned
Through this survey, we sought to understand how automated tenant screening tech is used by landlords to screen renters in California—and how these opaque algorithms leave both renters and landlords in the dark. We then compiled and analyzed our findings into Screened Out of Housing. These are our key takeaways.
AI-enabled tenant screening systems are widely used in the rental market
Almost two-thirds of the landlords we surveyed received tenant screening reports that contained some AI-generated score or recommendation.
Our survey indicates that a significant percentage of landlords are making decisions without receiving individualized information on the applicant. When asked what they receive from their tenant screening vendors, 10% of landlords reported receiving only a risk score, and 28% reported receiving only a recommendation. That means that 38% of landlords rely on unvalidated third-party screening analysis to make rental decisions.
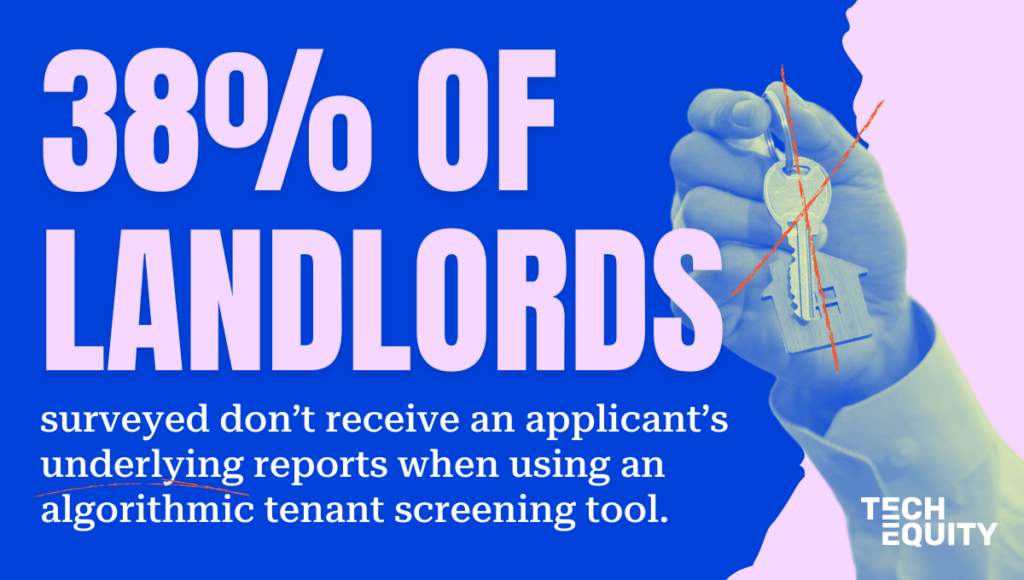
What’s more, about 37% of landlords said that they follow what screening companies say without any additional discretion.
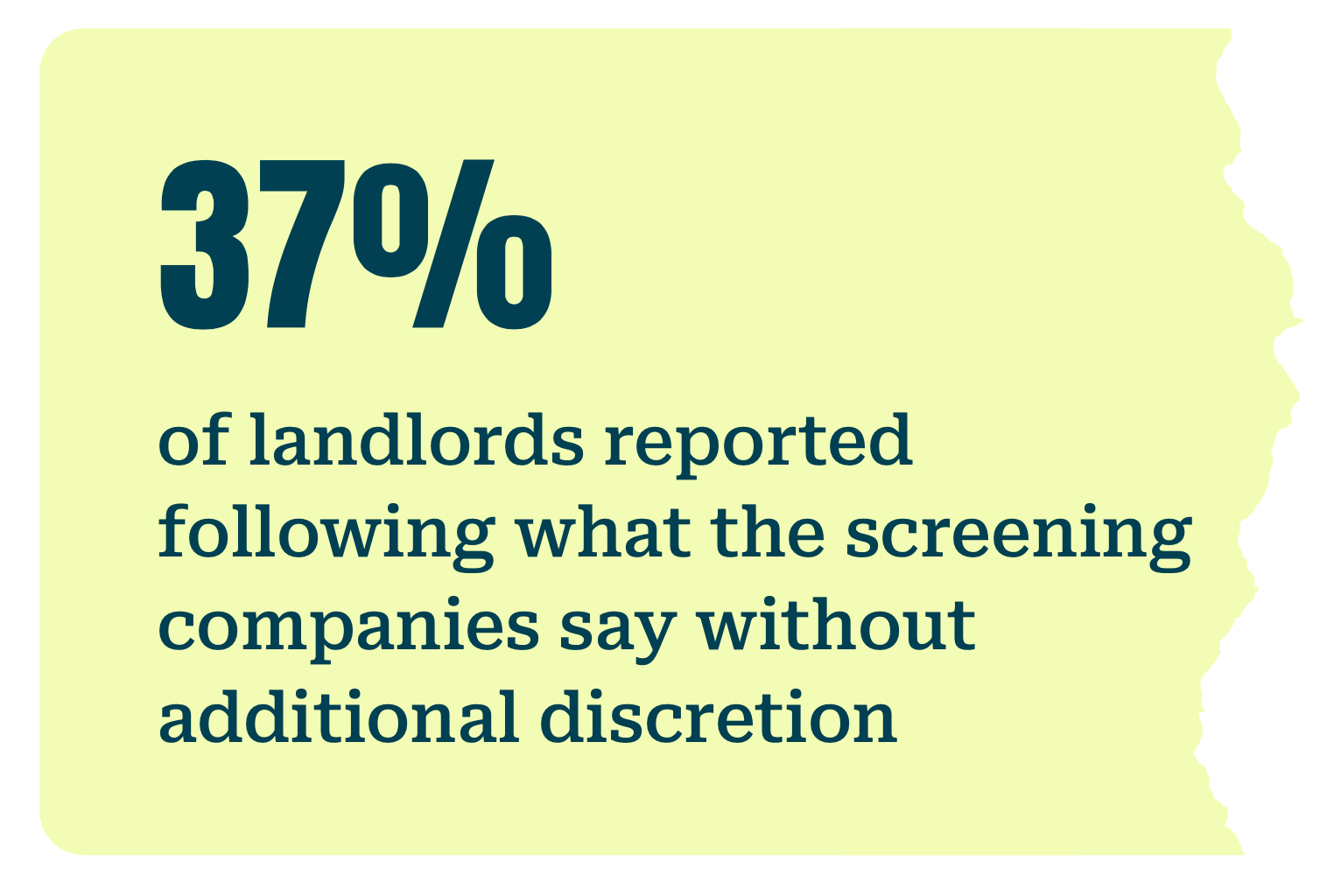
The use of Minority Report-esque predictive scoring for renters is prevalent
Nearly one in five landlords reported receiving predictive information from screening companies. These analytics give landlords predictions about how likely a potential renter is to pay rent late, break their lease early, or even whether or not they’ll damage the property. This sort of predictive analysis, which judges people based on actions they have not yet taken, was recently made partially illegal in Europe.
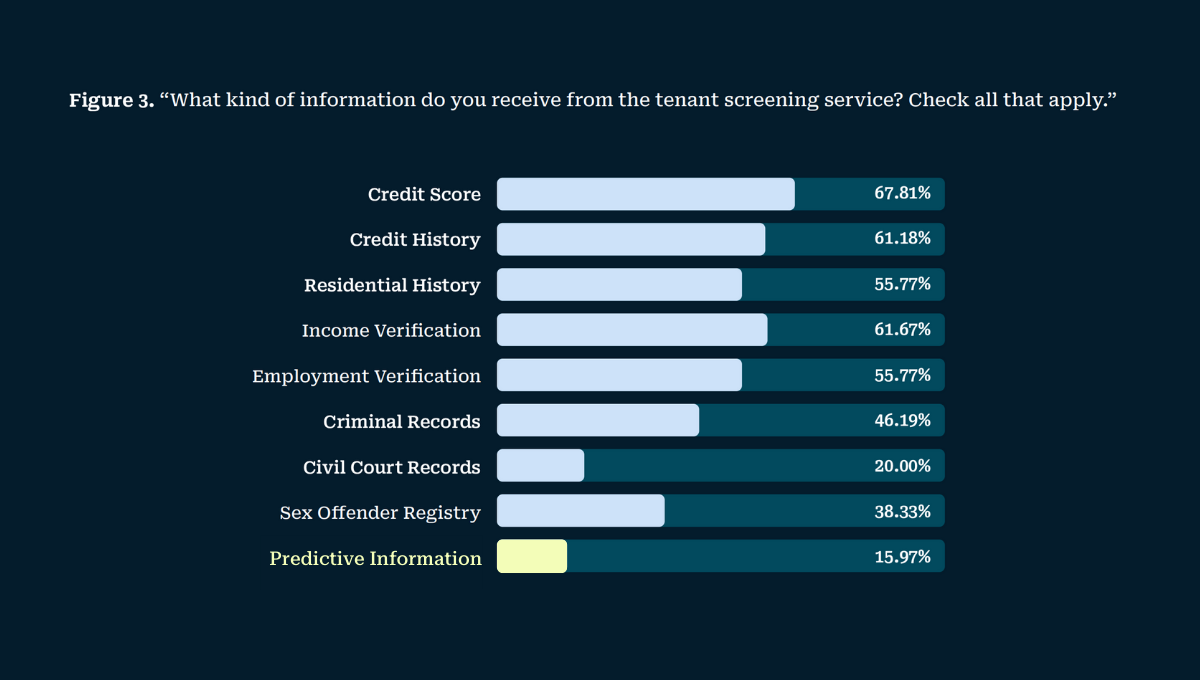
Renters are often left in the dark, deepening power imbalances that threaten housing rights
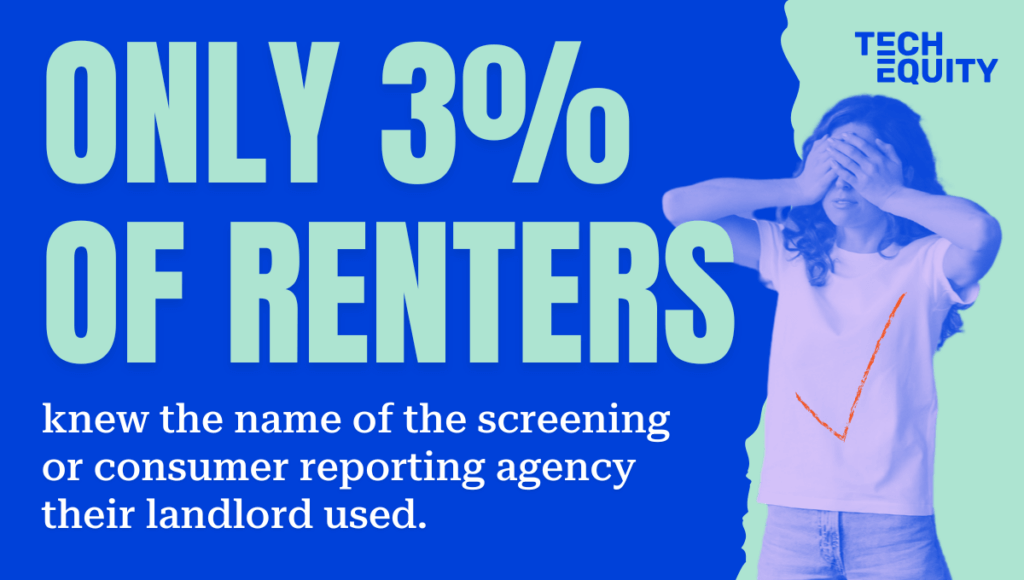
Only 3% provided the name of a screening or consumer reporting agency; the rest left the response blank or erroneously provided the name of their landlord or property management company.
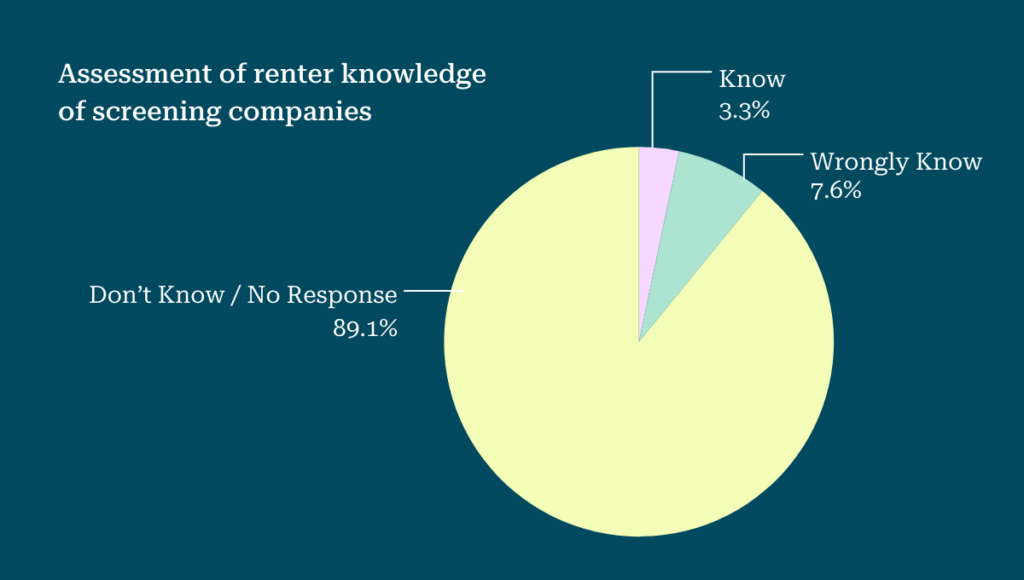
This raises the question: how can renters enforce their rights if they don’t know who is screening them and how they’re doing it?
Companies are amassing troves of data on renters— yet renters themselves, their advocates, and even landlords are operating in the dark. Protections including the Fair Housing Act (FHA) and Fair Credit Reporting Act (FCRA) govern the rental application process to ensure fairness and nondiscrimination, but if renters are not aware of all parties involved in the decision, they are starting from behind to enforce their rights.The structural transparency issues enable discrimination to go undetected in the shadows, and once again leave renters to pick up the slack of a broken system.
AI tenant screening systems disproportionately impact the most vulnerable renters
We found that landlords who serve lower-income renters and smaller landlords used AI-enabled tenant screening the most. Landlords operating 1-4 units were 57% of the total sample, but 62% of those who reported relying on automated recommendations for rental decisions.
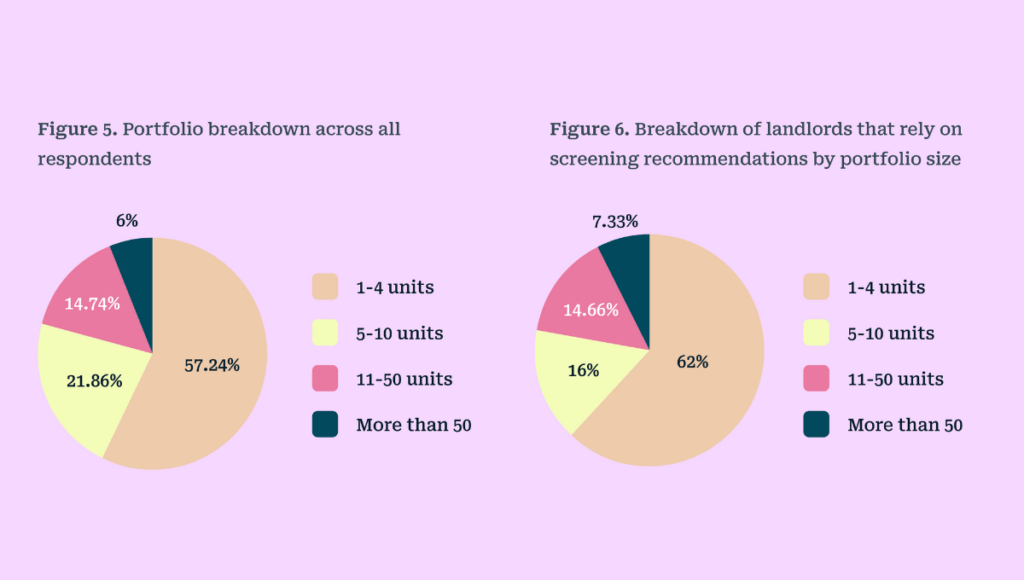
What’s more, landlords charging lower rents are more likely to rely on algorithmic recommendations alone than landlords overall.
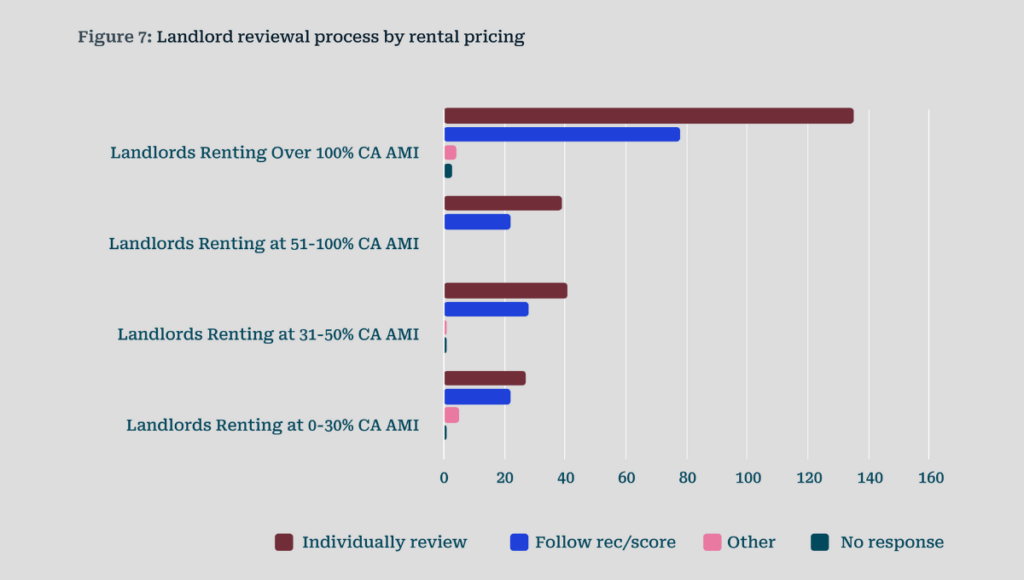
Some of our most vulnerable renters are essentially being used as test subjects for AI screening. This raises questions about potential bias, exploitation, and further financial marginalization.
This survey backs up what we know to be true – the current housing market is steeped in racial bias
Black and Latinx renter survey respondents were almost half as likely to have their rental applications accepted as white respondents (46% and 43%, respectively).
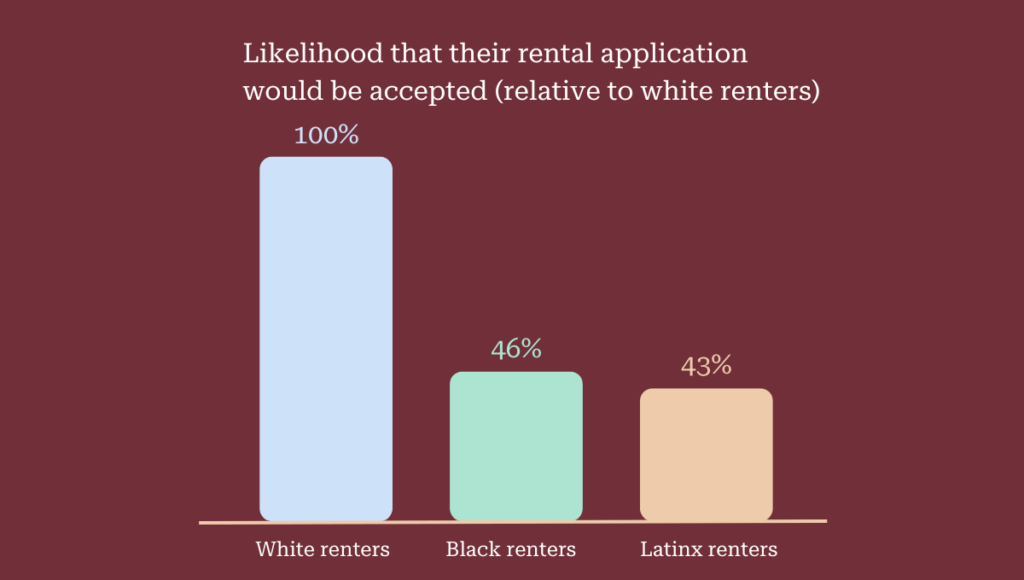
The overrepresentation of Black and Latinx renters across denied applications, as well as the overreliance for small portfolio and 0-30% AMI landlords, suggest that tenant screening AI is having an acute effect on vulnerable renters with the fewest housing options and legal protections.
Corrections Note: A previous version of this report had miscalculated figures relating to the information received by landlords: the highlighted statistic on page 15, Figure 3 on page 15, and Figure M on page 38 of the paper have all been updated with accurate calculations as of March 6th, 2025.
The path forward
AI’s role in housing is widespread and insidious. We’re already seeing automated tenant screening play a huge role in the California rental market, disproportionately impacting vulnerable renters. Renters need real transparency into how decisions about their housing options are made—and in some cases, new rights and rules to ensure that these tools do not further inequity in our housing system.
HUD’s recent guidance on the use of artificial intelligence in tenant screening offers common-sense recommendations for how landlords and screening companies must apply the Fair Housing Act to tenant screening in the age of AI, and how advocates and policymakers can hold the creators of these tools accountable.
We need this guidance to be codified into law for it to materially benefit renters. What’s more, we need to encode new laws that tackle the unique impact of automated tenant screening tools.
Shine light on tenant screening practices so renters and landlords aren’t left in the dark
With over a third of surveyed landlords relying on scores and recommendations without the underlying data behind them, there is a clear need to bring transparency to the tenant screening industry. We need screening companies to provide transparency into their algorithms and the recommendations they make. We need landlords to receive informed consent from renters for the use of this technology, and to share all information with consenting applicants.
Stop housing AI from deepening racial and economic inequities in the housing market
Algorithms can be biased in two main ways: from the data they’re fed and how they digest that data. We need to develop systems to make sure that tenant screening algorithms are not only accurate but are also accountable for discriminatory outcomes.
Make sure companies and regulators are accountable for harms and responsible for upholding renter protections, rather than leaving the burden on individuals
Right now, too much onus is put on renters to protect themselves against discrimination and bias—with very little power or transparency granted to them. The burden of accountability should be put on the shoulders of the companies that are making these housing decisions—and the regulators that are responsible for upholding our civil, consumer, and housing rights frameworks.
Want to learn more?
Check out the full paper here for more insights and policy recommendations. Want to get involved in building equitable housing AI policy? Sign up here to join us.